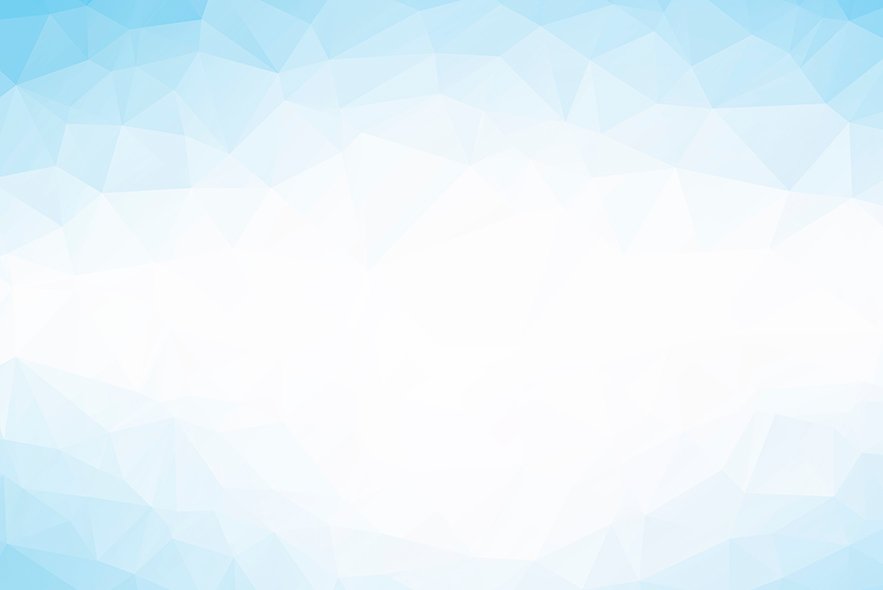
DevOps engineer
What is a DevOps engineer?
A DevOps engineer is an IT professional who works with software developers, systems operators and other production IT staff to oversee code releases and deployments. The role calls for someone who has relevant hard and soft skills to overcome the traditional barriers between software development, quality assurance, testing and IT operations teams and foster a collaborative, holistic environment.
Traditionally, the roles of software developers and IT operations staff were siloed and highly isolated. This meant that software was developed with little, if any, insight into the operations resources needed to host the application. When a software release candidate was ready for deployment, it was formally handed off to IT. IT was responsible for understanding the software's resource and performance requirements and then deploying, configuring and managing that workload in production.
This yielded an onerous and inefficient system where IT had to grapple with the needs and capabilities of software they knew little or nothing about, supported only indirectly by software project teams who handled bug reports and help tickets. This all occurred while IT worked on a subsequent patch or iteration of the product, which they had no knowledge or input about. When an update arrived from the development team, this slow and painful cycle simply repeated itself, handing off the new code to IT and letting Operations deal with deployment and its aftermath.
The rise of DevOps practices sought to overcome these technical and cultural barriers by overlapping the software development process with IT operations tasks. DevOps not only facilitates a faster and more iterative approach to software development and the software development lifecycle, it also lets developers provision, deploy, configure and manage each release in production with IT operations staff support as needed.
A DevOps engineer is the professional embodiment of this vital technical role which encompasses a broad scope of coding, testing, project management and operations abilities.
Roles and responsibilities of a DevOps engineer
A DevOps engineer must know how to manage the IT infrastructure that supports software code in dedicated, multitenant or hybrid cloud computing environments. They might have to provision resources, select an appropriate deployment model, direct testing to validate each release and monitor performance after release. Tasks might include test data preparation, results analysis, problem troubleshooting and issue communication back to the software developers.
The DevOps approach to software development aims for frequent, incremental changes to code versions, which means frequent deployment and testing regimens. Although DevOps engineers rarely code from scratch, they must understand the basics of software development languages and be familiar with the development tools used to create new code or update existing code. A DevOps engineer works with development staff to tackle the necessary coding and scripting to connect various application elements, including APIs, libraries and software development kits, and integrate other components such as Structured Query Language data management or messaging tools their teams need to run the software release on operating systems and production infrastructure.
Specifically, a DevOps engineer focuses on automation and maintenance in the live environment, so it's common to find job roles that underscore automation and maintenance of software products, systems and services. For example, a DevOps engineer might perform the following tasks:
- Automate using continuous integration and continuous delivery (CI/CD) in workflows.
- Perform on-call service for the system availability.
- Implement and maintain security through risk assessment and vulnerability management.
- Develop or source application and system management tools that mitigate manual effort.
- Implement automated management features, such as performance monitoring, diagnostics and failover and availability capabilities.
- Evaluate risks for all changes while maintaining high availability within the environment.
DevOps skills, training and certifications
The DevOps market is expected to grow considerably in the coming years and DevOps engineer positions will continue to be in high demand. According to Global Market Insights, the DevOps market was $8 billion (USD) in 2022 and is projected to grow to $70 billion by 2032.
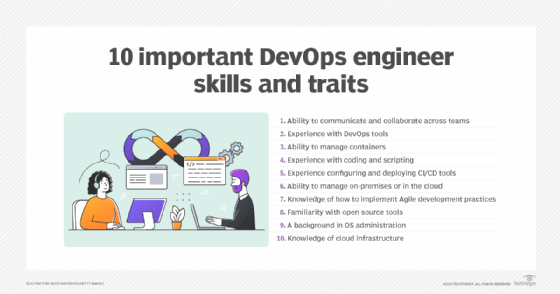
The role of DevOps engineer doesn't fall along one career track; professionals evolve into the position from a variety of backgrounds. For example, a DevOps engineer might start as a software developer who oversees aspects of IT operations. Conversely, a DevOps engineer might move up from a system administrator role after they've gained knowledge about coding, scripting, integration and testing. There can be some overlap between DevOps and SysOps engineer job descriptions, depending on the organization and its technical needs, but it's the DevOps engineer whose responsibilities include changing business processes as needed to solve organizational problems and improve business outcomes.
There are a variety of technical training offerings an IT professional can pursue to earn a DevOps certification. These range from introductory courses for those who are new to DevOps to advanced training courses for those who wish to become lead DevOps engineers. There are also courses specific to the DevOps tools an organization already uses as well as a broad range of emerging technologies. A variety of DevOps courses and certifications are also available for popular DevOps tools -- such as Chef, Puppet or Jenkins -- or the major cloud platforms.
Continuous testing can add significant value to software development. Learn how continuous testing in DevOps works and several best practices for its use.