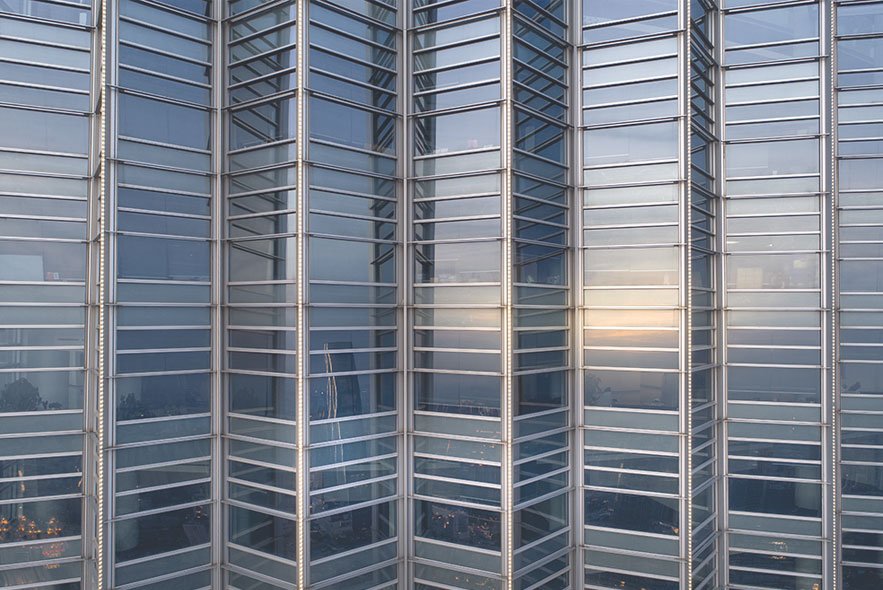
data integration
What is data integration?
Data integration is the process of combining data from multiple source systems to create unified sets of information for both operational and analytical uses. It's one of the core elements of the overall data management process -- the main objective of data integration is to produce consolidated data sets that are clean and consistent and meet the information needs of different end users in an organization.
Integrated data is fed into transaction processing systems to drive business applications and into data warehouses and data lakes to support business intelligence (BI), enterprise reporting and advanced analytics. Various data integration methods have been developed for different types of uses, including batch integration jobs run at scheduled intervals and real-time integration done on a continuous basis.
The importance and business benefits of data integration
Most organizations have numerous data sources, often including both internal and external ones. In many cases, business applications and operational workers need to access data from different sources to complete transactions and other tasks. For example, an online order entry system requires data from customer, product inventory and logistics databases to process orders; call center agents must be able to see the same combination of data to resolve issues for customers.
Loan officers have to check account records, credit histories, property values and other data before approving mortgages. Financial traders need to keep an eye on incoming streams of market data from internal systems and external sources. Pipeline operators and plant managers depend on data from various sensors to monitor equipment. In these and other applications, data integration automatically pulls together the necessary data for users so they don't have to combine it manually.
It's the same in BI and analytics systems: Data integration gives data analysts, corporate executives and business managers a comprehensive view of key performance indicators (KPIs), customers, manufacturing and supply chain operations, regulatory compliance, business risks and other aspects of business processes. As a result, they have better information available for tracking business performance, managing operations, planning marketing campaigns and other tasks.
By bringing together data sets for these purposes, effective data integration provides the following business benefits to organizations:
- Higher-quality data for business decision-making. Data integration ensures that business executives and workers get relevant data to help drive business decisions. It often also includes data cleansing and other data quality measures to fix errors, inconsistencies and other issues in data sets.
- Easier access to data for analytics. Integrating data in a data warehouse or another centralized repository makes it easier for data scientists, other analysts and self-service BI users to access the data they're looking to analyze. Required data can also be delivered directly to them through integration-driven data pipelines.
- Fewer data silos. Data integration can help break down departmental data silos that lock potentially useful data away from users in other parts of an organization.
- Increased efficiency for data users. Because data integration reduces the work that users have to do to find the data they need, they can devote more time to their real jobs.
- More data-driven business operations. Overall, data integration helps organizations become more data-driven in their strategic planning and operational decision-making.
How data integration works
At a basic level, data integration connects source and target systems and routes data from the former to the latter. In some cases, the actual data is moved to a target system, as in real-time integration of different data streams. In others, copies of data sets from source systems are fed into a target one -- for example, when transactional data is consolidated in a data warehouse or smaller data mart for analysis.
From a technical standpoint, data integration architects and developers create software programs that automate and manage the process of integrating data sets. Some forms of data integration are relatively straightforward -- replicating data from one system to another is a case in point. Often, though, different database schemas in separate source systems need to be harmonized as part of integration projects.
One common approach for doing so is to create a mediated schema that merges local source schemas into a global one and then use data mapping to bridge the differences between them and match data elements to the mediated schema. That can be done in a target system, such as a data warehouse, or in a virtual architecture that creates a unified view of data from different systems without physically loading it into a new repository.
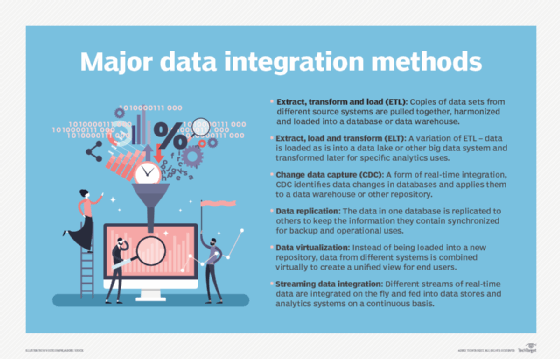
Types of data integration
The most prevalent data integration method is extract, transform and load (ETL), which is commonly used in data warehousing. In ETL jobs, data is extracted from source systems and run through a data transformation process to consolidate and filter it for analytics uses; the resulting data sets are then loaded into a data warehouse. ETL is a batch process that typically involves bulk amounts of data, and it can also be used to feed varied sets of big data into Hadoop clusters and other data lake platforms.
However, an alternative extract, load and transform (ELT) method is often used in big data systems. ELT inverts the second and third steps of the ETL process, loading raw data into a target system and then filtering and transforming it as needed for individual analytics applications. That's a popular option for data scientists, who often do their own data preparation work and want to have access to full data sets for predictive modeling, machine learning applications and other advanced analytics uses.
Forms of real-time data integration include change data capture (CDC), which applies updates made to the data in source systems to data warehouses and other repositories, and streaming data integration, which integrates real-time data streams and feeds the combined data sets into databases for operational and analytical uses. Another data integration method that can be done in either real time or batch mode is data replication, which copies data from one data source to another system to synchronize them for operational, backup and disaster recovery (DR) purposes.
Data integration methods also include data virtualization, which evolved from an earlier approach known as data federation. It uses a virtual data layer to integrate data instead of doing so physically. That gives business users and data analysts an integrated view of different data sets without requiring an IT team to load the data into a data warehouse, an operational database or another target system. Data virtualization can augment an existing analytics architecture for specific applications or be used as part of a logical data warehouse or data lake environment that includes a mix of different platforms.
Data integration use cases and examples
These are some of the foremost use cases for data integration in organizations:
Feeding data into data warehouses, data lakes and other repositories. The process of creating and updating analytics data stores relies heavily on data integration work. That includes the data warehouses and data lakes that generally support different kinds of analytics applications: basic BI and reporting by the former vs. data science and advanced analytics by the latter. A newer option is the data lakehouse, which merges elements of data lakes and data warehouses. For all three, data sets from different source systems are loaded into the repository and often combined with one another.
Creating data pipelines. Data integration also plays a key role in the process of building data pipelines that deliver data to users for both operational and analytics uses. Many pipelines pull data from integrated systems and applications to provide the required information.
Integrating customer data. This involves consolidating data about customers from all available sources, including contact details, account records, customer lifetime value (CLV) scores and information collected through customer service calls, website visits, surveys, social media posts and other interactions. Doing so gives companies a current and complete view of customers, which can help them better target their marketing efforts and identify opportunities to cross-sell and upsell customers on products. Well-integrated customer data can also improve customer service by giving call center workers and field service technicians immediate access to the information they need.
Streamlining BI, analytics and data science applications. Data integration initiatives typically also pull together data on revenues, expenses, profits, productivity and other KPIs from across an organization. That information is then made available to corporate and business executives in reports or BI dashboards to help improve both operational management and strategic planning. Similarly, data science teams can do advanced analytics work more quickly if data is integrated for them upfront.
Making big data more accessible and usable. Big data environments often include a combination of structured, unstructured and semistructured data. In the past, much of that data went unused, but storing, processing and using it is more feasible now because of data lakes and data lakehouses. As a resulting, integrating sets of big data has become a priority in many organizations.
Processing IoT data. Increasingly, organizations are moving to integrate data collected by multiple sensors installed on industrial equipment, including manufacturing machines, vehicles, elevators, pipelines, electrical grids, oil rigs and other devices connected to the internet of things (IoT). Integrated sets of sensor data can be used to monitor operations and run predictive maintenance models that aim to identify potential equipment failures before they occur, which can help reduce unplanned downtime for repairs.
Data integration challenges
Common challenges that data management teams encounter on data integration include the following:
- Keeping up with growing data volumes.
- Unifying inconsistent data silos.
- Dealing with the increasingly broad array of databases and other data platforms in IT infrastructures.
- Integrating cloud and on-premises data.
- Resolving data quality issues.
- Handling the number of systems that need to be integrated and their distributed nature, especially in large organizations with global operations.
The amount of data being generated and collected by organizations creates particularly big integration challenges. Data volumes continue to grow quickly, and the rate of that growth is only likely to increase as big data applications expand, the use of low-cost cloud object storage services rises and IoT develops further. With so much data involved, successfully planning and managing the required data integration work is a complicated process.
Data integration tools and techniques
Developers can hand-code data integration jobs, typically in the form of scripts written in Structured Query Language (SQL), the standard programming language used in relational databases. For many years, that was the most common approach to integration. But packaged data integration tools that automate, streamline and document the development process have become available from various IT vendors. Open source integration tools are also available, some free and others in commercial versions.
Prominent data integration vendors include the following companies, as well as others:
- AWS.
- Boomi.
- Cloud Software Group's IBI and Tibco Software units.
- Google Cloud.
- Hitachi Vantara.
- IBM.
- Informatica.
- Microsoft.
- Oracle.
- Precisely.
- Qlik.
- SAP.
- SAS Institute.
- Software AG.
- Talend.
ETL tools were among the first data integration software products, reflecting the ETL method's central role in the data warehouse systems that emerged in the mid-1990s. Now, many vendors offer more expansive data integration platforms that also support ELT, CDC, data replication, big data integration and other integration methods; in addition, associated data quality, data catalog and data governance software is often included as part of the platforms.
Some of the integration platform vendors provide data virtualization tools, too. They're also available from data virtualization specialists and other data management vendors, including AtScale, Data Virtuality, Denodo Technologies, IBM's Red Hat unit and Stone Bond Technologies.
The growth of cloud computing has created new needs for organizations to integrate data in different cloud applications and between cloud and on-premises systems. That led to the development of integration platform as a service (iPaaS), a product category that provides cloud-based integration tools. Most of the major data integration platform vendors now also offer iPaaS technologies; other companies in the iPaaS market include Jitterbit, Salesforce's MuleSoft unit, SnapLogic and Workato.
Data integration best practices for businesses
The following are some best practices to adopt as part of data integration initiatives.
Document your data architecture and IT systems. Data managers and data integration developers need full documentation of the source and target systems in an organization's data architecture so they can do the required mapping between them. They also must have a solid understanding of both internal and external data sources, the business rules that are embedded in the data, and how often data is updated and modified.
Work collaboratively with business units and departments. To accomplish the above, data management teams must work closely with business users. For example, data modeling with input from business users is a key step in documenting data requirements for applications; a data model also diagrams how data needs to flow between applications and systems. In addition, close ties with the business helps teams choose the right integration methods to use for different applications.
Link integration work with other data management processes. Data integration efforts should be aligned with data governance programs, as well as related data quality and master data management (MDM) initiatives. Doing so helps ensure that data is clean and consistent and that data lineage information is available to help integration developers better understand what's in data sets.