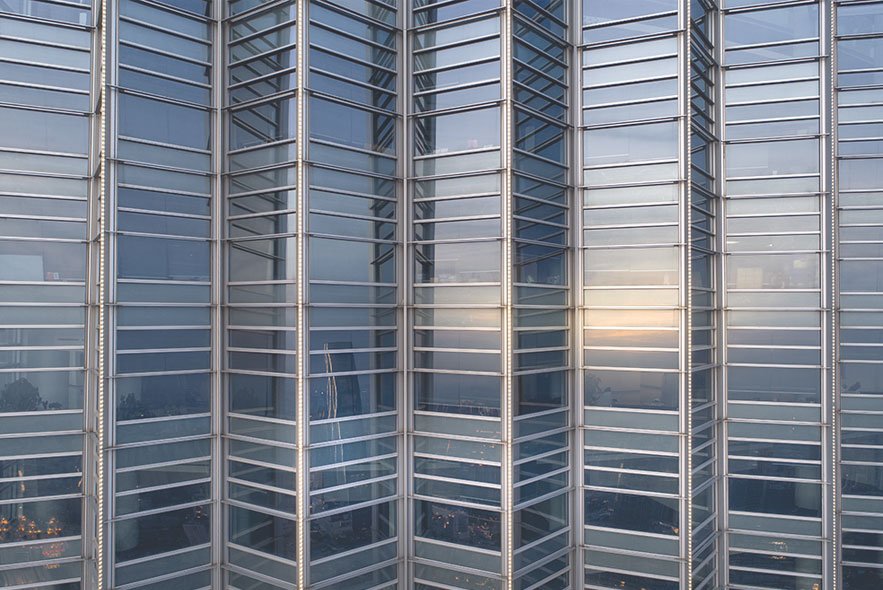
data warehouse
A data warehouse is a repository of data from an organization's operational systems and other sources that supports analytics applications to help drive business decision-making. Data warehousing is a key part of an overall data management strategy: The data stored in data warehouses is processed and organized for analysis by business analysts, executives, data scientists and other users.
Typically, a data warehouse is a relational database or columnar database housed on a computer system in an on-premises data center or, increasingly, the cloud. Data from online transaction processing (OLTP) applications and additional internal or external sources is extracted and consolidated in the data warehouse for business intelligence (BI) uses that include ad hoc querying, decision support and enterprise reporting. Users access the data through BI software and other types of analytics tools.
Basic components of a data warehouse architecture
At a fundamental level, a data warehouse architecture contains a set of three tiers that include the following main components:
- A database server for processing, storing and managing data as the bottom tier.
- An analytics engine that runs BI applications and queries as the middle tier.
- BI and reporting tools that support data analysis, visualization and presentation as the top tier.
An architecture commonly also contains a data integration layer with tools that extract and combine data from operational systems and a staging area where data is cleansed, transformed and organized before being loaded into the data warehouse. A combination of data integration and data quality software is used to carry out the tasks at the staging level.
An enterprise data warehouse stores analytical data for all of an organization's business operations; alternatively, individual business units may have their own data warehouses, particularly in large companies. Data warehouses can also be connected to multiple data marts, which are smaller systems containing subsets of an organization's data for a specific department or group of users.
Data warehouses also support online analytical processing (OLAP) technologies, which organize data into cubes categorized by different dimensions to help accelerate the analysis process. In addition, the data records stored in a data warehouse contain detailed metadata and summary data to help make them searchable and useful to business users.
Types of data warehouses and deployment options
There are two main approaches to implementing a data warehouse: the top-down method and the bottom-up method.
The top-down method was created by data warehouse pioneer William H. Inmon. It calls for building the enterprise data warehouse first and then using the data stored in it to set up data marts for business units and departments. Under Inmon's approach, data is extracted from source systems and validated in a staging area before being integrated into a normalized data model and transformed for planned BI and analytics uses.
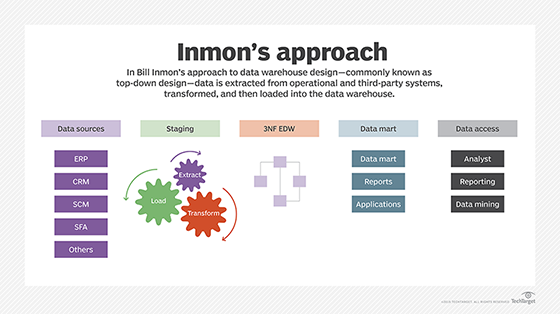
The bottom-up method was developed by consultant Ralph Kimball as an alternative data warehousing approach that calls for dimensional data marts to be created first. Data is extracted from sources and modeled into a star schema design, with one or more fact tables connected to one or more dimensional tables. The data is then processed and loaded into data marts, which can be integrated with one another or used to populate an enterprise data warehouse.
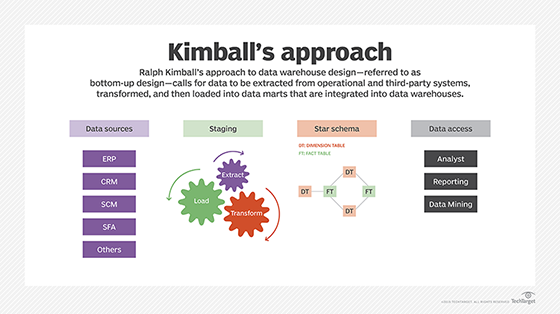
There is also a hybrid approach to data warehouse design that includes aspects of both the top-down and bottom-up methods. Organizations that adopt a hybrid strategy often seek to combine the development speed of the bottom-up approach with the data integration capabilities that can be achieved in a top-down design.
Finally, some organizations have adopted federated data warehouses that integrate separate analytical systems already put in place independently of one another -- an approach that proponents describe as a practical way to take advantage of existing deployments.
Data warehouse benefits
Data warehouses can benefit organizations from both a business and IT perspective. For example, potential benefits include the following:
- By consolidating data from different sources, data warehouses give corporate executives and other decision-makers a more comprehensive view of business operations, performance and trends than they could easily get otherwise.
- Data warehouses also offer increased data quality and consistency for analytics uses, thereby improving the accuracy of BI applications.
- Managed and used effectively, data warehouses enable more-informed business decisions that can help organizations improve productivity and operational efficiency, increase revenue and gain competitive advantages over business rivals.
- The information generated from data warehouses can be used to respond more proactively to market trends, business problems and new business needs.
- Data warehouses provide a better understanding of customer behavior and preferences to help organizations improve marketing, sales and customer service -- and, ultimately, customer satisfaction.
- Separating analytical processes from operational ones by moving them to a data warehouse can enhance the performance of operational systems and enable data analysts and business users to access and query relevant data faster.
- To best meet their business and IT needs, organizations can choose between on-premises systems, conventional cloud deployments and data warehouse as a service (DWaaS) offerings that free them from the need to deploy, configure and administer data warehouses.
Common data warehouse use cases and applications
Because a data warehouse enables faster and more efficient access to various data sets and typically has the compute and memory resources needed to run complicated queries, it can help businesses quickly derive insights and, thus, value from their data. To help meet business objectives, data warehouses are commonly used for the following purposes:
- Single source of truth. Many organizations, especially larger ones, have a complex operational structure, with various business units and departments each producing significant amounts of data. That data can be collected, integrated and merged in a data warehouse, giving users more complete data sets for more accurate insights and better business decisions. In doing so, an organization can break down data silos that limit access to some data sets and often result in inconsistent data. The data warehouse instead becomes a single source of truth for the organization as a whole, ensuring users in different units work with the same data.
- Reporting. Similarly, a data warehouse can consolidate various types of data, including current operational data and historical data -- some of which previously might have been locked away in legacy systems. As a result, it holds in one location all the data needed for internal reporting to business executives and managers. Moreover, the data warehouse enables the speedy delivery of that information -- reports often can be generated and distributed in seconds or minutes versus hours or days if they are assembled from data in different systems.
- Data mining. Data mining is the process of sorting through large data sets to identify patterns and relationships that can provide insights into future trends and inform decision-making. Although newer data lake platforms that hold varied sets of big data are now often used for data mining in advanced analytics applications, the data warehouse continues to support data mining as part of BI applications in many organizations.
- BI and AI. Operational databases typically can't handle the processing required for BI querying and artificial intelligence (AI) initiatives, but data warehouses can. They typically can also deliver the large amounts of data BI and AI applications require for optimal accuracy and -- in the case of machine learning and natural language processing, in particular -- successful learning.
- Auditing and regulatory compliance. The data warehouse, with its stores of historical data from across the enterprise, also supports audit and compliance processes. It can efficiently provide relevant records, saving an organization time and money in its audits and regulatory compliance work while also eliminating errors and enabling more accurate data analysis.
Best practices for designing and managing a data warehouse
Here are some best practices to adopt as part of the data warehouse design and management process.
Understand the business goals and strategies that drive the need for a data warehouse. The data warehouse holds data that's structured and processed so it's ready for analytical queries. That's why it's important to start by first understanding the organization's data warehousing needs and the business reasons behind them. IT leaders and data management teams should involve business stakeholders in these discussions, as the business objectives driving the need for the data warehouse will help shape decisions on the data to include, required data sources and how to format data sets.
Review the organization's data governance program, data management plan and supporting processes. The value of a data warehouse stems from the data it holds, not the underlying hardware and software. As a result, an organization should review its data governance program and overall data management strategy and update them if needed to support the data warehouse's planned business use cases. Data management processes at all the designated source systems should also be reviewed to help ensure the data being fed into the data warehouse is clean, accurate and consistent.
This planning should also consider how often data needs to be loaded and whether to go with batch or real-time data processing, based on the business use cases. Additionally, project managers and their teams should confirm that there's an ongoing process for revisiting such considerations and updating data management plans and processes. For example, this step might include deploying change data capture capabilities, so that any changes made in databases are replicated in the data warehouse. Teams need to define user permissions and access controls and address broader data security and compliance requirements upfront, too.
Select the right data warehouse architecture, platform and tools. Business use cases and requirements should also be considered to determine the right technologies for the data warehouse. There's a host of questions to ask. For example, do business requirements necessitate an on-premises data warehouse or would a cloud-based one better meet the organization's objectives? Also, should extract, transform and load (ETL) tools or the alternative extract, load and transform (ELT) method be used to feed data into the warehouse? Should there be a source-agnostic integration layer? Should the organization deploy a data warehouse platform itself or use a managed service?
Adopt new processes to optimize the data warehouse and maximize its business value. Established and emerging practices can help organizations optimize the management of a data warehouse and maximize the value it delivers. For example, data observability techniques can aid in maintaining the health of data in enterprise systems and data pipelines. Applying Agile development methodologies to data warehouse management can make it possible to deliver business value more quickly and with lower risks than using a traditional waterfall approach. Enabling self-service BI and analytics capabilities can similarly speed up value delivery by making it easier for business users to analyze data themselves.
On-premises vs. cloud data warehouses
Like other types of IT systems, data warehouses increasingly are moving to the cloud. All major data warehouse vendors now offer cloud-based systems that are available both for conventional user-managed deployments and as part of fully managed DWaaS offerings. They include vendors that first offered on-premises data warehouses -- most notably, IBM, Microsoft, Oracle, SAP and Teradata -- as well as ones that developed data warehouses specifically for the cloud, such as Amazon Web Services (AWS), Google and Snowflake.
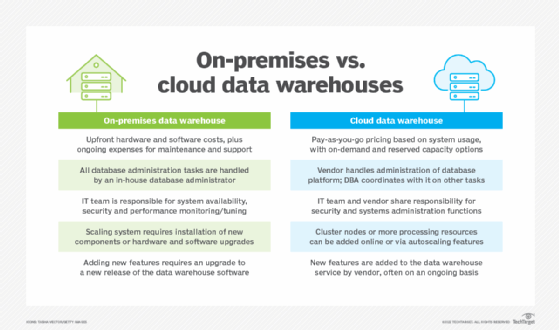
In general, the underlying architecture is the same in on-premises and cloud data warehouses. But they both come with potential benefits and drawbacks in comparison to one another. For example, a data warehouse running on an on-premises server can have the following advantages over a cloud-based one:
- Faster response time and less latency for users.
- More control of database administration, system maintenance and security by IT and data management teams.
- Tighter oversight for regulatory compliance purposes, especially in highly regulated industries.
- Potentially lower -- or at least comparable -- costs over the lifetime of the data warehouse, even if upfront costs are higher because of the need to buy and install new technology.
On the other hand, cloud data warehouses offer the following advantages:
- Security, data backup and disaster recovery capabilities provided by the cloud vendor, particularly in DWaaS environments.
- Easier and faster scalability as data volumes and system resource needs grow.
- Lower startup costs, with no upfront hardware or software purchases required.
- Faster installation and provisioning of a new data warehouse.
- Potentially higher reliability, as cloud providers generally offer more redundancy and have deeper data management skills than most organizations can afford on their own.
- Easier connections to other cloud-based services than an on-premises data warehouse typically can provide.
Another option is a hybrid data warehouse environment that combines cloud and on-premises systems. For example, an organization could keep sensitive data in an on-premises data warehouse for data privacy and regulatory compliance reasons, while moving other data sets to a cloud-based repository.
Data warehouses vs. data lakes vs. databases
Data lakes and data warehouses both support analytics applications, but there are notable differences between the two data repositories. Data warehouses typically store processed data in predefined schemas designed for specific BI, analytics and reporting applications. Usually, they hold conventional structured data from transaction processing systems and other business applications.
By contrast, a data lake is a repository for all types of raw data, whether it's structured, unstructured or semistructured. Data lakes are most commonly built on Hadoop, Spark or other big data platforms, not databases. A schema doesn't need to be defined upfront in a data lake -- instead, data sets can be analyzed as is or filtered and prepared for individual analytics applications. ELT processes are common in data lakes, vs. the ETL approaches most often used in data warehouses.
As a result of their flexibility and support for varied data sets, data lakes can handle more advanced types of analytics than data warehouses. For example, they can be used for machine learning, predictive modeling, text mining and other data science applications.
Data warehouses also differ from operational databases. While a data warehouse stores data from multiple sources for analysis, an operational database is generally used to collect, process and store data from a single system or application to support ongoing business processes. The data in such databases is then consolidated, cleansed and fed into data warehouses.
Data warehouse history and innovations
The concept of data warehousing can be traced back to work conducted in the mid-1980s by IBM researchers Barry Devlin and Paul Murphy. The duo coined the term business data warehouse in their 1988 paper, "An architecture for a business and information system," which described the framework of an information retrieval and reporting system built for use within IBM. The paper stated:
"The architecture is based on the assumption that such a service runs against a repository of all required business information that is known as the Business Data Warehouse (BDW). ... A necessary prerequisite for the physical implementation of a business data warehouse service is a business process and information architecture that defines (1) the reporting flow between functions and (2) the data required."
Bill Inmon, as he is more familiarly known, furthered data warehouse development with his 1992 book Building the Data Warehouse, as well as by writing some of the first columns about the topic. As part of his top-down design methodology for creating a data warehouse, Inmon described the technology as a subject-oriented, integrated, time-variant and non-volatile collection of data that supports an organization's decision-making process.
The technology's growth continued with the founding in 1995 of The Data Warehousing Institute, an education and research organization now known as TDWI that focuses more broadly on data analytics and management technologies. That was followed by the 1996 publication of Ralph Kimball's book The Data Warehouse Toolkit, which introduced his dimensional modeling and bottom-up approach to data warehouse design.
Early adopters began deploying data warehouses in the mid-1990s, and mainstream usage by organizations began to grow later that decade and became widespread in the 2000s. In 2008, Inmon updated his methodology by introducing a Data Warehouse 2.0 concept that focused on the inclusion of data lifecycle management capabilities, unstructured data, metadata and compartmentalized processing environments.
The next big evolution of the data warehouse came in 2012, when AWS launched the first cloud-based data warehouse, Amazon Redshift. Other vendors soon followed suit, and the number of cloud data warehouse options proliferated in subsequent years. Vendors have also layered more automation and intelligence features into their data warehouse software. For example, Oracle in 2018 released Oracle Autonomous Data Warehouse, a version of its Autonomous Database technology that the company describes as "self-driving."
In addition, big data systems have become a valuable extension of data warehouses in many organizations. In some cases, Hadoop clusters or other big data platforms serve as a staging area for traditional data warehouses. In others, data warehouses and data lakes are deployed in a unified analytics environment.
That also has led to the development of the data lakehouse, which combines a data lake's flexibility and scalability with the querying and data management features of a data warehouse. The concept was first outlined in 2017, and data lakehouse technologies have become available from various vendors since then.