Building a big data architecture: Core components, best practices
To process the infinite volume and variety of data collected from multiple sources, most enterprises need to get with the program and build a multilayered big data architecture.
The volume and variety of structured, semistructured and unstructured data growing exponentially and generated at increasingly high velocities from a broad range of sources is the essence of big data.
Researchers have estimated that the world will create 463 exabytes of data daily by 2025 -- that's 463 billion gigabytes per day.
No one enterprise, of course, will need all that data. But they'll need to collect, store and analyze as much of it as possible to gain an edge from the actionable insights required to effectively compete and succeed in this digital age.
Yet many organizations are only starting their big data journey. "Most enterprises are just scratching the surface of what big data can do," said Christophe Antoine, vice president of global solutions engineering at data integration platform provider Talend. A major reason: They don't have a big data architecture in place. "If you're just replicating what you've been doing," Antoine added, "there's a big chance you'll be unhappy with the results."
What is a big data architecture?
Businesses need to evolve their technology stack to handle the volume and variety of data available to them, and they need to implement the infrastructure capable of doing that work at top speed -- often in real or near real time.
"Traditional databases and data processing technologies have not been able to scale to meet the demands of enterprises," said Sripathi Jagannathan, general manager of data engineering and platforms at digital transformation service UST.
That's where a big data architecture comes in. It's purposefully designed to ingest, process and analyze data too large or too complex for traditional database systems to handle. Moreover, it's intended to scale as an enterprise's big data program grows -- both in the amount of data being used as well as the number of business use cases that depend on the organization's big data.
"Big data architecture," Jagannathan explained, "is an approach to infrastructure and software that facilitates the storage and processing of really large volumes and variety of data being generated at varying velocities."
Big data architecture components
IT advisors said they have seen some business leaders mistakenly seek out a one-shot solution to serve their big data ambitions. In reality, organizations need to design and implement a multilayered architecture to successfully handle the full range of tasks required within a big data program.
The simplest big data architecture model features three layers, said Sandhya Balakrishnan, U.S. region head at big data analytics consultancy Brillio.
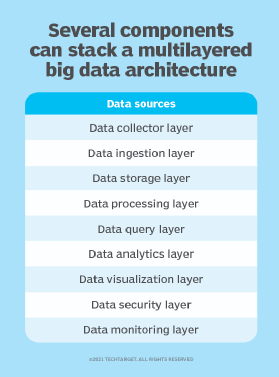
The storage layer holds the data being ingested by the organization from the various data generating sources -- whether those sources are the enterprise's own operational systems, third-party systems or other endpoints.
The processing layer could perform batch processing, real-time processing or hybrid processing.
The consumption layer lets an organization use data in various ways through analytics engines, data queries, or AI and machine learning applications and encompasses data visualization, which can be enabled by a host of different tools.
Other models break down a big data architecture into more layers, separating out more individual components. Some models, for example, list the separate layers as follows: data collector, data ingestion, storage, processing, data query, data analytics and data visualization.
Other models further add data security and data monitoring.
Design and deployment challenges
IT teams typically encounter multiple challenges as they design and deploy their big data architecture and manage all the supporting infrastructure:
- Selecting the right components and tools that balance current requirements, future needs, costs and expected returns.
- Integrating the various components -- particularly integrating with legacy systems that generate data -- to collect, process and make use of all the required data.
- Collecting, integrating and processing at the speed and scale required by an enterprise's use cases.
- Having the necessary skills to adequately assess options, develop and mature the architecture, and ultimately manage the deployed technology. "You have to have architects who know exactly the pros and cons and have experience on why to use one [technology] over another one," Antoine noted.
- Ensuring that the data is secure based on regulatory requirements, privacy standards and best practices.
- Enabling trust in the data so that users are confident in the results produced by maximizing the data's value. "You have to build the right utility and tools to make sure the data quality is visible," Balakrishnan said.
- Optimizing the data. "We can store, transport, clean, query and present data in many ways," said Jeremiah Cunningham, data science engineer at life insurance provider Bestow. "The business may choose to investigate data under different slices -- perhaps time-based, cohort-based or some more complex sub-population. [But] accessing large amounts of data in complex ways creates an optimization problem that can manifest in different forms."
Big data architecture best practices
Experienced technology leaders offered the following best practices for designing and operating a big data architecture that can deliver results:
- Develop a nuanced view of the business value that the organization wants to achieve with its big data program and use that assessment to guide an agile delivery of the needed technologies.
- Build the architecture according to the strategic vision and approach it as an agile program while creating enough templates to make it scalable. "Build the technology foundation with a holistic view," Balakrishnan advised.
- Decouple systems to "ensure new tools and technologies can be integrated without significant disruption," Jagannathan said.
- Create a robust data governance program to ensure that the data is well secured, complete for the planned use cases and trusted by users.
Examples of available technologies
Numerous technologies come together to form a big data architecture, so enterprise IT architects can select tools from multiple vendors when deploying their infrastructure.
The estimated value for the global big data market is projected to grow nearly 20% annually and surpass $243 billion by 2027, according to the report "Big Data - Global Market Trajectory & Analytics" by Research and Markets. Technologies that make up the big data ecosystem include the following:
- extract, transform and load tools;
- data lakes and data warehouses;
- cloud platforms for processing and storage;
- business intelligence and data visualization software; and
- data governance and data security tools.
"Investigate the current needs and future prospects of data at your organization and construct a plan of what conceptual technologies you need," Cunningham advised. "By researching potential products and choices early, you can reduce the problem space into a manageable size and then get input from your peers on potential problems or unexpected gains. [It's critical] to properly investigate and select the right technologies for the right job to maximize productivity while minimizing time and costs."